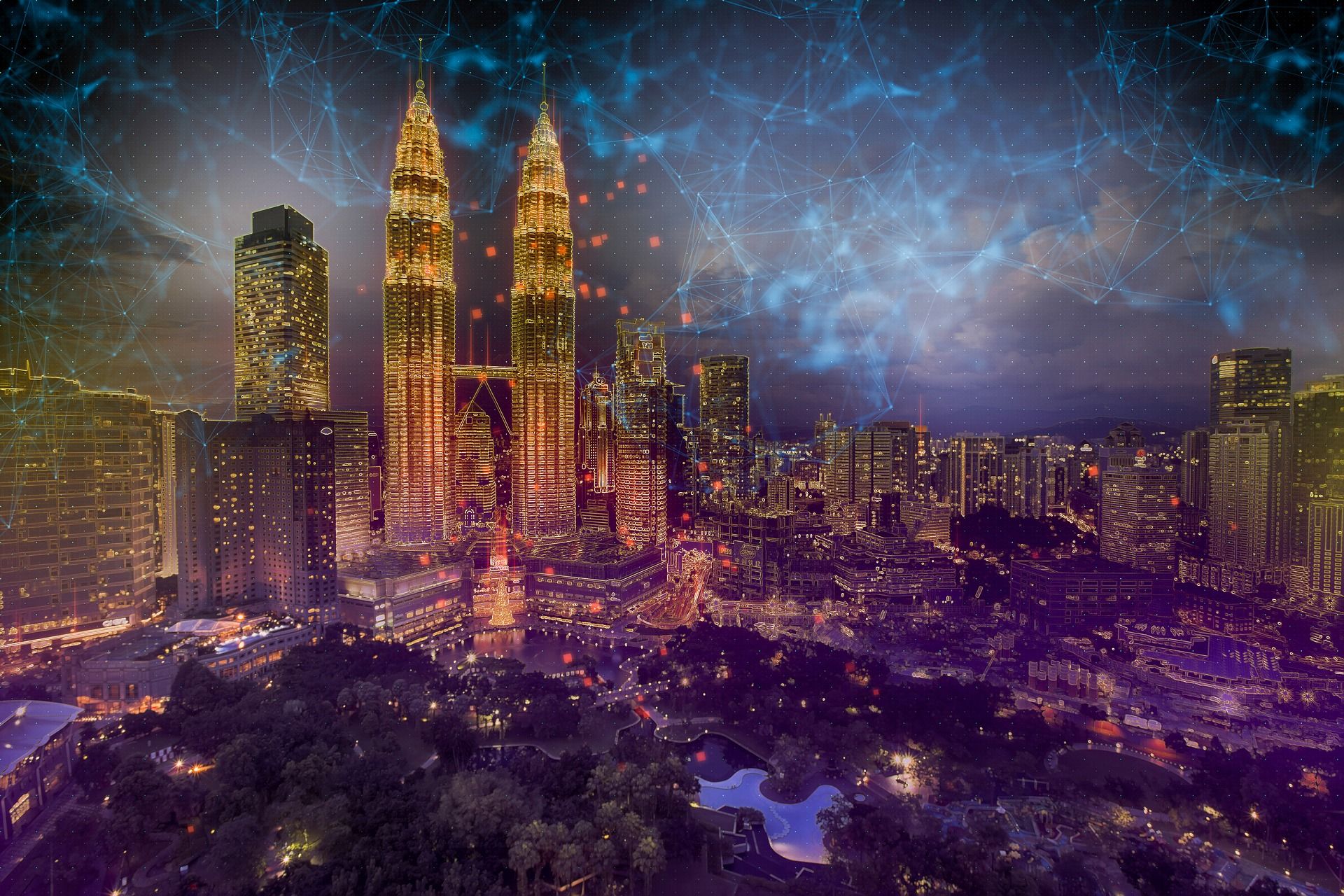
Cities are complex systems and managing cities has shown to be an equally complex job. Harvesting data regarding the built environment, social aspects and economics in the city is a way to make better-informed decisions. A new conceptual approach to the way this data is organized and used has been called recently “digital twin”, borrowing the term used for a longer time in some fields of the industry, such as aerospatial and automotive.
According to Grieves (2014), a digital twin can be considered “a mirror image of a physical process that is articulated alongside the process in question, usually matching exactly the operation of the physical process which takes place in real time”. From regional geographic information systems into building operation and asset management, data comes from plenty of different sources when a model is twinning a built environment.
The known British professor, Michael Batty (2018) claims that the idealization of having digital twins and reality as identical twins will never be achieved, but the main quest in city modelling would be to link the functional environment (i.e. human behaviour, preferences) and the physical urban environment to socio-economic processes in cities. For Batty, there are three different types of digital twins, also called families of simulation models for urban analytics:
- A physical representation of the elements that define the buildings in the city – building in digital representation, VR, AR and thence BIM. The Virtual London Platform, ViLo, is one example of twin in this sense.
- Flow of traffic which is a problem in demand and supply in term time. This could be extended to real time dynamic data in general, or the high frequency city as Batty calls it. The simulations undertaken by London’s metropolitan transportation authority in order to improve service would be one instance of this kind of twin.
- A model of the low frequency city simulating long term urban growth and change. The QUANT model, for example, uses spatial analytics to reconstruct and preview phenomena such as the impact of wages in housing prices at the regional scale.
Figure 1: Smart City Diagram (Batty, 2020).
Castelli et al (2019) from the Italian National Research Council developed the Urban Intelligence (UI) paradigm, which proposes a framework defined by a structure for the digital component of the city, defined as the cyber-physical counterpart of all the city systems and subsystems. The main characteristics of its architecture is: full multidisciplinary integration of city layers, constant connection and evolution, integration of participative strategies to include human-oriented information and modularity of application.
Figure 2: Proposed architecture for Urban Intelligence. Source: Castelli et al (2019).
Figure 2 above illustrates the UI architecture proposed by Castelli et al (2019). This paradigm aims at creating a unified multidisciplinary approach, rather than solving specific problems. The items in this architecture are further explained below:
- City Knowledge Models: The design and development of the city model takes place in the start-up phase of the platform construction: capitalizing on data provided by cartography, laser scans and other methods, data layers of interest can be collected and indexed, making them easily accessible by services in the UI platform. The 3D data is used beyond visualization, since simulations and numerical methods may require knowledge about the entities, like using them as boundary conditions, for example. It must be precise enough so metrics can be extracted automatically from the 3D model, requiring no physical measurements. Metrics for evaluating the status of each relevant city sub-system can be defined along with computational methods to evaluate them on the digital twin.
- Data Collection: This module is designed to acquire data arising from (a) the network of sensors, mainly densely deployed low-energy devices, (b) participatory tools such as apps and questionnaires to get input from citizens and (c) intelligent actuators acting on the city system according to the solutions design by the other modules of the platform.
- Data Lake and ICT Platform: An ICT platform that must store, manage, access and analyze the data collected. The data lake concept refers to organizing a large amount of data (in native format, formatted or output of processes) for documentation, visualization, data analytics and knowledge acquisition. It has the data as a static component and a dynamic component to provide the functionalities necessary to manage and exploit these data. The platform is useful to support the management of all the sub-systems of the urban organism.
- Modelling and Simulations: The sense-reason-act loops are exploited by different technologies to simulate the subsystems of the city, for example advanced machine learning, well-defined mathematical structures or more basic calculations. The idea is that due to the complexity of the city system, many different solutions can be adopted, developing a number of special purpose controllers.
- City Digital Twin and Decision-Making via Multidisciplinary Analysis and Optimization: Modeling and simulation tools provide data-driven, physics-based and assimilated models for each disciplinary domain. The Multidisciplinary Analysis (MDA) provides integration of all modules. The optimization is applied to double-proof the solutions, reconciling different approaches in coordinated efforts able to cope with heterogeneity. The core of the twinning process is achieved by integrating the City Knowledge Model with simulation tools.
As an effort to guide the development of digital twins for the built environment in the United Kingdom, the Centre for Digital Built Britain (2018) has launched the Gemini principles. They are listed in Figure 3, organized under the pillars of purpose, trust and function. These key values intend to enable a further connection of digital twins creating a National Digital Twin (NDT). The NDT will not be a huge singular model, but an ecosystem of digital twins connected via securely shared data, which requires an information management framework. And not even all digital twins would be connected, but only where this connection generates added value. As they can be connected in numerous ways, the ecosystem would consist of different federations of digital twins, getting more diverse and interconnected over time.
Figure 3: The Gemini Principles. Source: Centre for Digital Built Britain (2018).
The Centre for Digital Built Britain (2018) also recognizes the variety within the ecosystem of digital twins, classifying this diversity in the following aspects:
- Variety of purposes: (a) potential futures, such as strategic planning and simulation of proposed scenarios; (b) current state, like intervention management and real-time status monitoring and control; (c) record-keeping to enable learning from the past.
- Variety of spatial scales: (a) asset or building scale; (b) network or neighborhood scale; (c) system, city or regional scale; (d) national scale.
- Variety of temporal scales: (a) operational timescale; (b) reactive maintenance timescale; (c) planned maintenance timescale; (d) capital investment timescale.
- Variety of approaches to modelling: (a) geometric and geospatial modelling; (b) computational/mathematical/numerical modelling; (c) artificial intelligence and machine learning.
For Griffith & Truelove (2021), a digital twin is composed by some generic components defined as follows: (i) data, that can be spatial, statistical or streamed from IoT devices; (ii) data services, which transform and prepare data sources so they are fit for purpose for the use in a digital twin platform, like converting to different file formats, making data accessible or providing access to authorized users; (iii) platforms/applications enabling visualization, analytics or workflow, supporting decision and control systems and (iv) use, where digital services are consumed by the end users. The authors also established some categories of roles that entities can play in the digital twin ecosystem. An organization or group of organizations can occupy one or more of these roles depending on its expertise, institutional mission or legal jurisdiction:
a) digital twin platform/application operator: responsible for developing, operating and maintaining a digital twin platform or application.
b) digital twin data service provider: provides digital twin data services to data custodians or digital twin platform operators.
c) digital twin data custodian: collects, generates, maintains data for the purpose of carrying out their functions.
d) digital twin data user: end-user of the digital twin platform, which are able to draw insights and decision support about issues of interest.
e) digital twin stakeholder: could be impacted by the digital twin, either in connection with the supply and use of data that could be personal or confidential.
Figure 4: Federated Spatial Digital Twin Functional Architecture. Source: Griffith & Truelove, 2021.
In spite of the variety of frameworks for the overall architecture of digital twins, all of them count somehow with a material component, in this case the built environment, and a layer of abstractions or applications. While inspired by the existing conceptual approaches, there is no universal framework, since every digital twin will have its own architecture depending on the use cases and resources available. The time invested in thinking on the overall architecture beforehand instead of developing specific modules and applications right away can pay off while saving development time and taking advantage of existing commercial and open source solutions.