During my internship, I had the opportunity to explore the possibilities and limitations of City3D: a 3D Building Reconstruction program developed by TUDelft (Huang et al., 2022). City3D offers a fully automated process for reconstructing 3D buildings from raw airborne LiDAR point clouds and building footprints. With this, it addresses the common limitations of missing vertical walls and roof structure. Not to mention, the program is free software, meaning research can exploit it to create large-scale 3D building datasets. The Netherlands has already been making significant progress in creating LOD 2.2. 3D building models, like 3DBAG. However, similar datasets are not always readily available for other countries. Therefore, it was for me to assess whether City3D could help us in generating such datasets for countries like France, where comprehensive 3D building information may be lacking.
The model is based on a hypothesis-and-selection-based framework. This involves systematically assuming various potential outcomes, ultimately selecting the most truthful reconstruction. It bases its selection on simplicity, compactness, regularity, and good fitting to the input data. Unlike previous models, this approach claims to decrease user interaction and increase the usability for large-scale point clouds.
I explored the model by testing two study areas: A neighbourhood in Krimpen aan den Ijssel in the Netherlands and the village centre of Villard de Lans in France. For the Dutch dataset, I explored both the results of AHN data and google 3D mesh data with OSM footprints. Although meshes are not generally supported by the model, I still wanted to explore the outcomes to see if this can be a solution for regions without airborne LiDAR point clouds. For the French dataset, I explored LIDAR HD data (Géoservices, n.d.) with OSM footprints.
Results
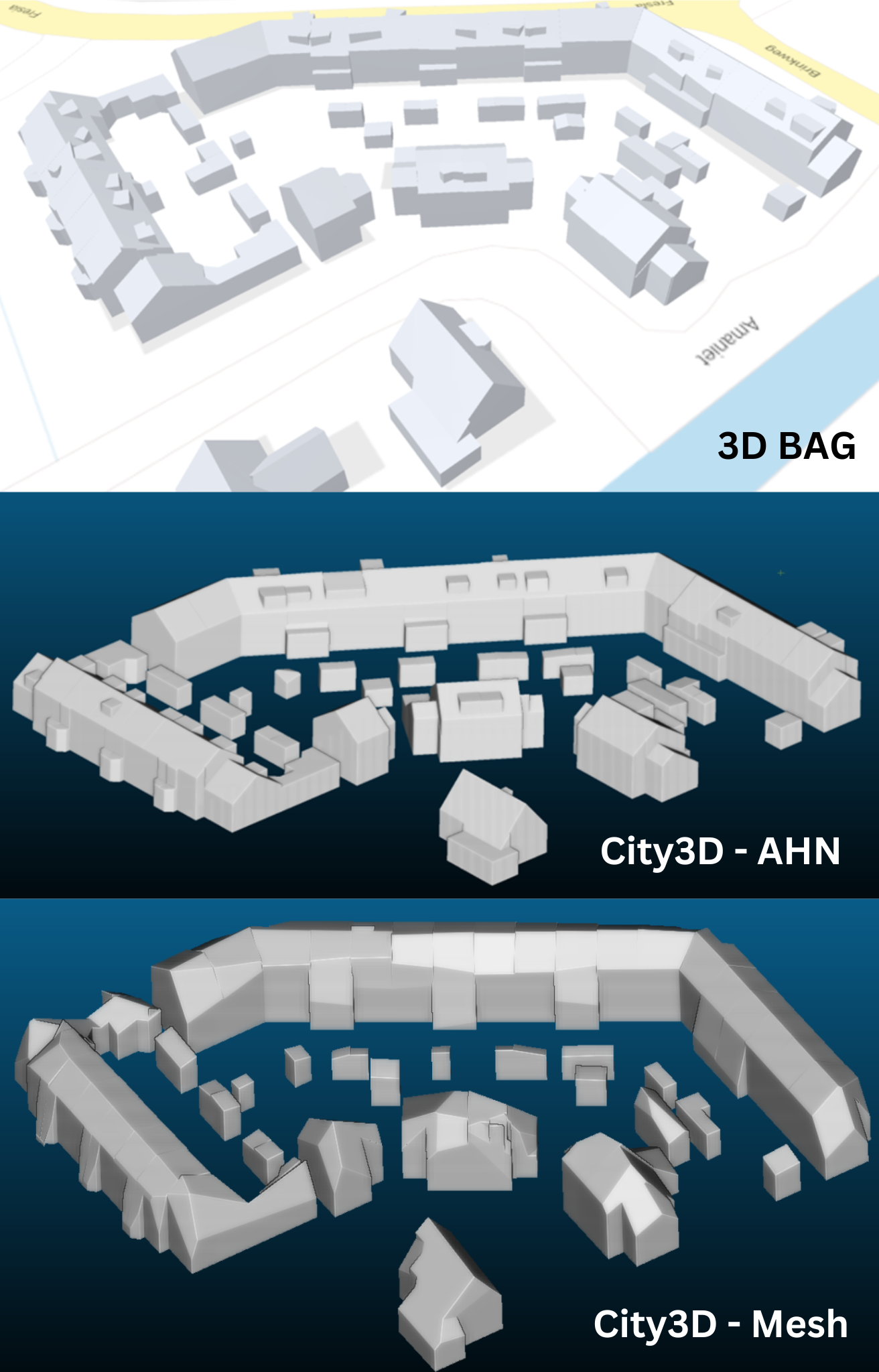
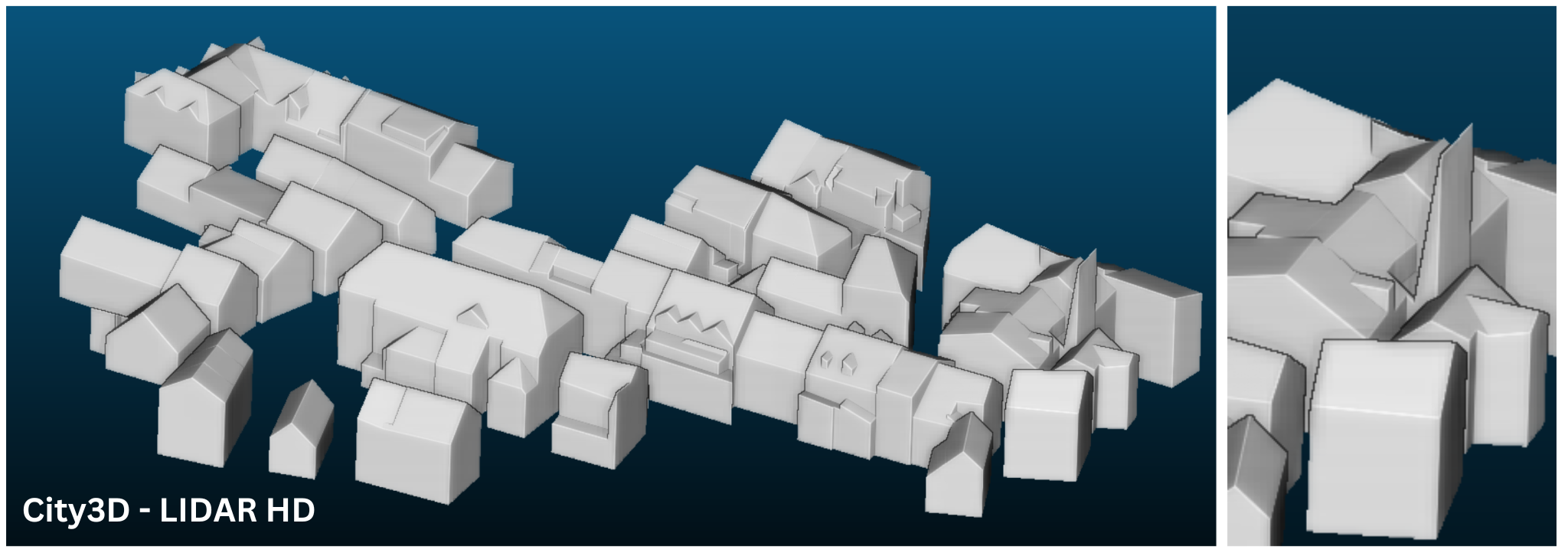
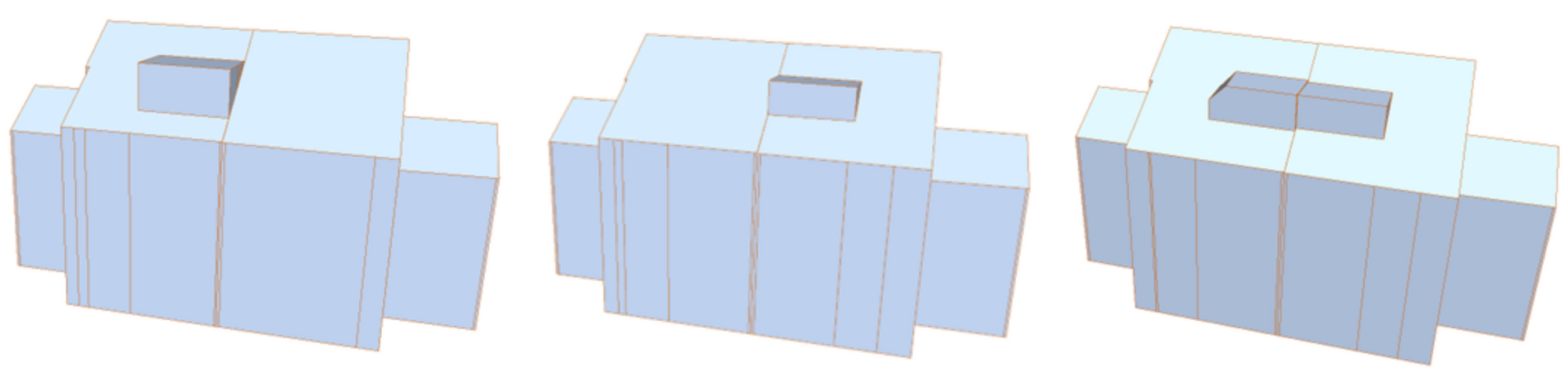
What’s next
City3D has great potential, offering an open-source opportunity for reconstructing LOD 2.2 3D buildings with notable precision. Particularly, it is a worthy starting point for 3D building constructions in countries such as France, that lack 3D building datasets like 3D BAG. City3D does come with some notable limitations and cumbersome workarounds that must be addressed before its full potential can be achieved.
To begin, the program's reliance on planar primitives poses a concern, as it compromises detail and accuracy in buildings with curved structures. While this is somewhat forgivable in a first iteration of LOD 2.2 3D buildings, it remains a limitation. In terms of structural regularity, City3D generally outperforms 3D BAG. However, it occasionally removes structural components due to excessive regularity control. Second, the flexibility of adjustable parameters proves beneficial, yet tweaking a parameter for improvement, typically leads to deteriorated results in another structural component. This implies that there is generally no optimal configuration. Moreover, the program's hypothesis-based approach always causes variability in the results without altering the parameters. Therefore, maintaining the same results for multiple datasets is not manageable.
Moving on to limitations of the general execution; City3D is currently compatible with only a limited number of input formats and some with rather weak support. For instance, the las format has to be translated due to large coordinate values, but this translation is subsequently required for the footprint input. However, instead of using the defined z-values of the footprint, the program simply finds the lowest point of the point cloud data and adds the footprint data to this height. Although this challenge is solvable, it requires additional work. Moreover, the absence of intermediate saves during the reconstruction process is a notable drawback, as errors in a single building can result in the loss of the entire reconstruction.
All things considered, the model has great potential to help reconstruct a large-scale dataset, such as for France. This is largely thanks to its high qualitative performance, especially compared to 3DBAG. Moreover, addressing the issues and improving the execution process would significantly enhance the program's usability and reliability.
References
Huang, J., Stoter, J., Peters, R., & Nan, L. (2022). City3D: Large-scale building reconstruction from airborne LiDAR point clouds. Remote Sensing, 14(9), 2254.
IGN France. (n.d.). LiDAR HD - Classées [Data set]. Géoservices, République Française. https://geoservices.ign.fr/lidarhd